Data Reconciliation
It is essential to have accurate and consistent data in a data-driven world. In data reconciliation, data from different sources is aligned and compared to ensure it is accurate and reliable. Our Data Reconciliation Services help businesses validate, correct, and harmonize their data for confident decision-making.

Our Data Reconciliation Services
Complere Infosystem provides the opportunities it presents—enhanced decision-making capabilities, improved customer experiences, and the ability to innovate more rapidly—make it a worthwhile endeavor for any data-driven organization.
We have over 200 data experts onboard and over 30 data projects in our portfolio.
Data Integration and Cleanup

Data Integration Strategies
Implementing efficient methods for reconciling data from multiple sources.

Data Cleansing
Removing or correcting erroneous data to improve overall data quality.
Data Reconciliation Tools

Technology Integration
Integrating reconciliation tools and software into your existing systems.

User Training
Equipping your team with the skills to utilize reconciliation tools effectively.
Monitoring and Maintenance

Ongoing Monitoring
Regularly checking for new discrepancies and addressing issues promptly.

Performance Optimization
Fine-tune reconciliation processes for maximum efficiency.
Data Assessment and Audit

Comprehensive Analysis
We start with a thorough assessment of your data to identify inconsistencies and discrepancies.

Data Quality Assessment
Evaluating data quality issues and their impact on your organization.
Data Migration

Data Assessment
We evaluate your existing data, ensuring that it's ready for migration by cleansing and formatting it as needed.

Data Migration Execution
Our experts handle the seamless transfer of your data to the new platform while preserving data integrity.
Platform Deployment

Efficient Deployment
We ensure a smooth deployment of the new platform, making sure all functionalities work as expected.

User Training
To maximize adoption, we offer user training to ensure your team is comfortable with the new platform.
Automated Reconciliation processes

Custom Reconciliation Algorithms
Developing tailored reconciliation algorithms to meet your unique needs.

Scheduled Reconciliation
Implementing automated reconciliation routines to maintain data quality over time.
How can you benefit from Data Reconciliation?
Ensures Data Accuracy
- Data reconciliation helps in identifying and rectifying discrepancies or inconsistencies in datasets. By comparing data from multiple sources, organizations can ensure that the data is accurate and reliable, leading to better decision-making processes.
Improves Operational Efficiency
- By automating the data reconciliation process, organizations can significantly reduce the time and effort required to verify data accuracy manually. This leads to improved operational efficiency and allows employees to focus on more strategic tasks, thus saving both time and money.
Enhances Regulatory Compliance
- Many industries are subject to strict regulatory requirements regarding data accuracy and integrity, such as finance and healthcare. Data reconciliation helps in complying with these regulations by ensuring that the data provided to regulatory bodies is accurate and consistent, reducing the risk of penalties or legal issues.
Facilitates Better Decision Making
- Reliable and accurate data is essential for making informed decisions. Data reconciliation provides a trustworthy dataset that decision-makers can rely on. By ensuring data accuracy, organizations can make better strategic decisions, leading to improved business outcomes and a competitive edge.
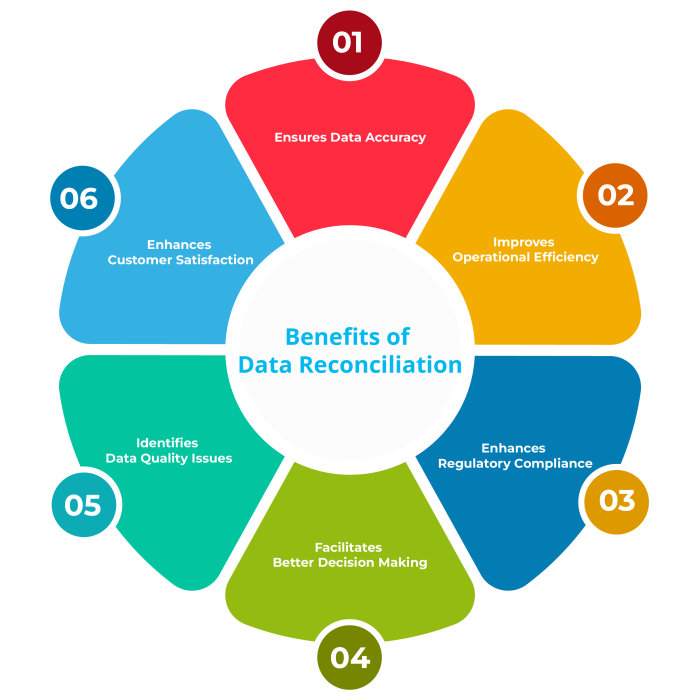
How it Works
01. Data Collection
-
Source Identification : Identify all the sources contributing to the data. Document the structure, format, and frequency of data from each source.
-
Data Extraction : Extract data from all identified sources using appropriate methods.Ensure that the extracted data includes all relevant fields required for reconciliation.
- Data Cleansing : Cleanse the extracted data to remove duplicates, inconsistencies, and errors. Standardize data formats, units, and terminology to ensure consistency.
03. Discrepancy Resolution
- Root Cause Analysis : Investigate the root causes of identified discrepancies. Determine whether discrepancies are due to data entry errors, system failures, or other factors.
-
Resolution Strategy : Develop a strategy to address and resolve discrepancies. Prioritize discrepancies based on their impact and criticality.
- Data Adjustment : Adjust data in the systems to rectify identified discrepancies. Ensure that adjustments are accurately documented and tracked.
02. Data Comparison
-
Matching Criteria Definition : Define criteria for matching records across different datasets. Establish rules for identifying and resolving discrepancies.
-
Data Alignment : Align data from different sources based on defined matching criteria. Use algorithms and tools to identify matching records and potential discrepancies.
- Discrepancy Identification : Identify discrepancies and inconsistencies between aligned datasets. Document all discrepancies, including their nature and potential impact.
04. Data Verification and Reporting
-
Verification Process : Verify the accuracy and consistency of reconciled data. Conduct thorough checks to ensure that all discrepancies have been resolved.
-
Documentation : Document the entire reconciliation process, including findings, actions taken, and adjustments made. Maintain comprehensive records for auditing and future reference.
- Reporting : Generate reports summarizing the reconciliation process and outcomes. Share reports with relevant stakeholders, including management, to ensure transparency and decision-making.
01. Strategy
- Clarification of the stakeholders’ vision and objectives
- Reviewing the environment and existing systems
- Measuring current capability and scalability
- Creating a risk management framework.
02. Discovery phase
- Defining client’s business needs
- Analysis of existing reports and ML models
- Review and documentation of existing data sources, and existing data connectors
- Estimation of the budget for the project and team composition.
- Data quality analysis
- Detailed analysis of metrics
- Logical design of data warehouse
- Logical design of ETL architecture
- Proposing several solutions with different tech stacks
- Building a prototype.
03. Development
- Physical design of databases and schemas
- Integration of data sources
- Development of ETL routines
- Data profiling
- Loading historical data into data warehouse
- Implementing data quality checks
- Data automation tuning
- Achieving DWH stability.
04. Ongoing support
- Fixing issues within the SLA
- Lowering storage and processing costs
- Small enhancement
- Supervision of systems
- Ongoing cost optimization
- Product support and fault elimination.
Why Choose Us for Data Reconciliation
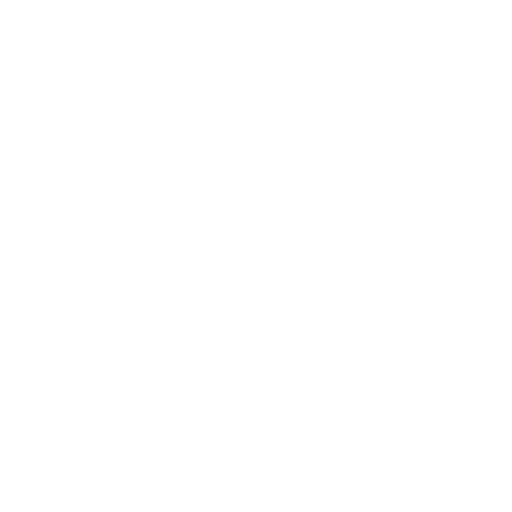
Expertise
Our team comprises skilled data reconciliation professionals with extensive industry experience.
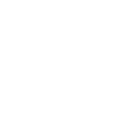
Custom Solutions
Tailored reconciliation strategies designed to meet your specific business needs.

Data Security
We prioritize data security and confidentiality throughout the reconciliation process.
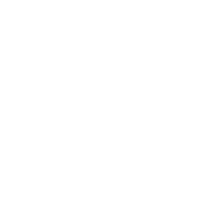
Cost-Effective
Reliable data reconciliation ensures accurate decision-making and reporting.